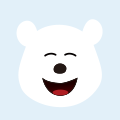
10分钟教你写一个数据库
今天教大家借助一款框架快速实现一个数据库,这个框架就是Calcite
,下面会带大家通过两个例子快速教会大家怎么实现,一个是可以通过 SQL 语句的方式可以直接查询文件内容,第二个是模拟 Mysql 查询功能,以及最后告诉大家怎么实现 SQL 查询 Kafka 数据。
Calcite
Calcite
是一个用于优化异构数据源的查询处理的可插拔基础框架(他是一个框架),可以将任意数据(Any data, Anywhere)DML 转换成基于 SQL 的 DML 引擎,并且我们可以选择性的使用它的部分功能。
Calcite能干什么
- 使用 SQL 访问内存中某个数据
- 使用 SQL 访问某个文件的数据
- 跨数据源的数据访问、聚合、排序等(例如 Mysql 和 Redis 数据源中的数据进行join)
当我们需要自建一个数据库的时候,数据可以为任何格式的,比如text、word、xml、mysql、es、csv、第三方接口数据等等,我们只有数据,我们想让这些数据支持 SQL 形式动态增删改查。
另外,像Hive、Drill、Flink、Phoenix 和 Storm 等项目中,数据处理系统都是使用 Calcite 来做 SQL 解析和查询优化,当然,还有部分用来构建自己的 JDBC driver。
名词解释
Token
就是将标准 SQL(可以理解为Mysql)关键词以及关键词之间的字符串截取出来,每一个token
,会被封装为一个SqlNode
,SqlNode
会衍生很多子类,比如Select
会被封装为SqlSelect
,当前 SqlNode
也能反解析为 SQL 文本。
RelDataTypeField
某个字段的名称和类型信息
RelDataType
多个 RelDataTypeField 组成了 RelDataType,可以理解为数据行
Table
一个完整的表的信息
Schema
所有元数据的组合,可以理解为一组 Table 或者库的概念
开始使用
1. 引入包
<dependency>
<groupId>org.apache.calcite</groupId>
<artifactId>calcite-core</artifactId>
<!-- 目前最新版本 2022-09-10日更新-->
<version>1.32.0</version>
</dependency>
2. 创建model.json文件和表结构csv
model.json 里面主要描述或者说告诉 Calcite
如何创建 Schema
,也就是告诉框架怎么创建出库。
{
"version": "1.0",//忽略
"defaultSchema": "CSV",//设置默认的schema
"schemas": [//可定义多个schema
{
"name": "CSV",//相当于namespace和上面的defaultSchema的值对应
"type": "custom",//写死
"factory": "csv.CsvSchemaFactory",//factory的类名必须是你自己实现的factory的包的全路径
"operand": { //这里可以传递自定义参数,最终会以map的形式传递给factory的operand参数
"directory": "csv"//directory代表calcite会在resources下面的csv目录下面读取所有的csv文件,factory创建的Schema会吧这些文件全部构建成Table,可以理解为读取数据文件的根目录,当然key的名称也不一定非得用directory,你可以随意指定
}
}
]
}
接下来还需要定义一个 csv
文件,用来定义表结构。
NAME:string,MONEY:string
aixiaoxian,10000万
xiaobai,10000万
adong,10000万
maomao,10000万
xixi,10000万
zizi,10000万
wuwu,10000万
kuku,10000万
整个项目的结构大概就是这样:
3. 实现Schema的工厂类
在上述文件中指定的包路径下去编写 CsvSchemaFactory
类,实现 SchemaFactory
接口,并且实现里面唯一的方法 create
方法,创建Schema
(库)。
public class CsvSchemaFactory implements SchemaFactory {
/**
* parentSchema 父节点,一般为root
* name 为model.json中定义的名字
* operand 为model.json中定于的数据,这里可以传递自定义参数
*
* @param parentSchema Parent schema
* @param name Name of this schema
* @param operand The "operand" JSON property
* @return
*/
@Override
public Schema create(SchemaPlus parentSchema, String name,
Map<String, Object> operand){
final String directory = (String) operand.get("directory");
File directoryFile = new File(directory);
return new CsvSchema(directoryFile, "scannable");
}
}
4. 自定义Schma类
有了 SchemaFactory
,接下来需要自定义 Schema
类。
自定义的 Schema
需要实现 Schema
接口,但是直接实现要实现的方法太多,我们去实现官方的 AbstractSchema
类,这样就只需要实现一个方法就行(如果有其他定制化需求可以实现原生接口)。
核心的逻辑就是createTableMap
方法,用于创建出 Table
表。
他会扫描指定的Resource
下面的所有 csv
文件,将每个文件映射成Table
对象,最终以map
形式返回,Schema
接口的其他几个方法会用到这个对象。
//实现这一个方法就行了
@Override
protected Map<String, Table> getTableMap(){
if (tableMap == null) {
tableMap = createTableMap();
}
return tableMap;
}
private Map<String, Table> createTableMap(){
// Look for files in the directory ending in ".csv"
final Source baseSource = Sources.of(directoryFile);
//会自动过滤掉非指定文件后缀的文件,我这里写的csv
File[] files = directoryFile.listFiles((dir, name) -> {
final String nameSansGz = trim(name, ".gz");
return nameSansGz.endsWith(".csv");
});
if (files == null) {
System.out.println("directory " + directoryFile + " not found");
files = new File[0];
}
// Build a map from table name to table; each file becomes a table.
final ImmutableMap.Builder<String, Table> builder = ImmutableMap.builder();
for (File file : files) {
Source source = Sources.of(file);
final Source sourceSansCsv = source.trimOrNull(".csv");
if (sourceSansCsv != null) {
final Table table = createTable(source);
builder.put(sourceSansCsv.relative(baseSource).path(), table);
}
}
return builder.build();
}
5. 自定义 Table
Schema
有了,并且数据文件 csv
也映射成 Table
了,一个 csv
文件对应一个 Table
。
接下来我们去自定义 Table
,自定义 Table
的核心是我们要定义字段的类型和名称,以及如何读取 csv
文件。
- 先获取数据类型和名称,即单表结构,从
csv
文件头中获取(当前文件头需要我们自己定义,包括规则我们也可以定制化)。
/**
* Base class for table that reads CSV files.
*/
public abstract class CsvTable extends AbstractTable {
protected final Source source;
protected final @Nullable RelProtoDataType protoRowType;
private @Nullable RelDataType rowType;
private @Nullable List<RelDataType> fieldTypes;
/**
* Creates a CsvTable.
*/
CsvTable(Source source, @Nullable RelProtoDataType protoRowType) {
this.source = source;
this.protoRowType = protoRowType;
}
/**
* 创建一个CsvTable,继承AbstractTable,需要实现里面的getRowType方法,此方法就是获取当前的表结构。
Table的类型有很多种,比如还有视图类型,AbstractTable类中帮我们默认实现了Table接口的一些方法,比如getJdbcTableType 方法,默认为Table类型,如果有其他定制化需求可直接实现Table接口。
和AbstractSchema很像
*/
@Override
public RelDataType getRowType(RelDataTypeFactory typeFactory){
if (protoRowType != null) {
return protoRowType.apply(typeFactory);
}
if (rowType == null) {
rowType = CsvEnumerator.deduceRowType((JavaTypeFactory) typeFactory, source,
null);
}
return rowType;
}
/**
* Returns the field types of this CSV table.
*/
public List<RelDataType> getFieldTypes(RelDataTypeFactory typeFactory){
if (fieldTypes == null) {
fieldTypes = new ArrayList<>();
CsvEnumerator.deduceRowType((JavaTypeFactory) typeFactory, source,
fieldTypes);
}
return fieldTypes;
}
public static RelDataType deduceRowType(JavaTypeFactory typeFactory,
Source source, @Nullable List<RelDataType> fieldTypes){
final List<RelDataType> types = new ArrayList<>();
final List<String> names = new ArrayList<>();
try (CSVReader reader = openCsv(source)) {
String[] strings = reader.readNext();
if (strings == null) {
strings = new String[]{"EmptyFileHasNoColumns:boolean"};
}
for (String string : strings) {
final String name;
final RelDataType fieldType;
//就是简单的读取字符串冒号前面是名称,冒号后面是类型
final int colon = string.indexOf(':');
if (colon >= 0) {
name = string.substring(0, colon);
String typeString = string.substring(colon + 1);
Matcher decimalMatcher = DECIMAL_TYPE_PATTERN.matcher(typeString);
if (decimalMatcher.matches()) {
int precision = Integer.parseInt(decimalMatcher.group(1));
int scale = Integer.parseInt(decimalMatcher.group(2));
fieldType = parseDecimalSqlType(typeFactory, precision, scale);
} else {
switch (typeString) {
case "string":
fieldType = toNullableRelDataType(typeFactory, SqlTypeName.VARCHAR);
break;
case "boolean":
fieldType = toNullableRelDataType(typeFactory, SqlTypeName.BOOLEAN);
break;
case "byte":
fieldType = toNullableRelDataType(typeFactory, SqlTypeName.TINYINT);
break;
case "char":
fieldType = toNullableRelDataType(typeFactory, SqlTypeName.CHAR);
break;
case "short":
fieldType = toNullableRelDataType(typeFactory, SqlTypeName.SMALLINT);
break;
case "int":
fieldType = toNullableRelDataType(typeFactory, SqlTypeName.INTEGER);
break;
case "long":
fieldType = toNullableRelDataType(typeFactory, SqlTypeName.BIGINT);
break;
case "float":
fieldType = toNullableRelDataType(typeFactory, SqlTypeName.REAL);
break;
case "double":
fieldType = toNullableRelDataType(typeFactory, SqlTypeName.DOUBLE);
break;
case "date":
fieldType = toNullableRelDataType(typeFactory, SqlTypeName.DATE);
break;
case "timestamp":
fieldType = toNullableRelDataType(typeFactory, SqlTypeName.TIMESTAMP);
break;
case "time":
fieldType = toNullableRelDataType(typeFactory, SqlTypeName.TIME);
break;
default:
LOGGER.warn(
"Found unknown type: {} in file: {} for column: {}. Will assume the type of "
+ "column is string.",
typeString, source.path(), name);
fieldType = toNullableRelDataType(typeFactory, SqlTypeName.VARCHAR);
break;
}
}
} else {
// 如果没定义,默认都是String类型,字段名称也是string
name = string;
fieldType = typeFactory.createSqlType(SqlTypeName.VARCHAR);
}
names.add(name);
types.add(fieldType);
if (fieldTypes != null) {
fieldTypes.add(fieldType);
}
}
} catch (IOException e) {
// ignore
}
if (names.isEmpty()) {
names.add("line");
types.add(typeFactory.createSqlType(SqlTypeName.VARCHAR));
}
return typeFactory.createStructType(Pair.zip(names, types));
}
}
- 获取文件中的数据,上面把
Table
的表结构字段名称和类型都获取到了以后,就剩最后一步了,获取文件中的数据。我们需要自定义一个类,实现ScannableTable
接口,并且实现里面唯一的方法scan
方法,其实本质上就是读文件,然后把文件的每一行的数据和上述获取的fileType
进行匹配。
@Override
public Enumerable<Object[]> scan(DataContext root) {
JavaTypeFactory typeFactory = root.getTypeFactory();
final List<RelDataType> fieldTypes = getFieldTypes(typeFactory);
final List<Integer> fields = ImmutableIntList.identity(fieldTypes.size());
final AtomicBoolean cancelFlag = DataContext.Variable.CANCEL_FLAG.get(root);
return new AbstractEnumerable<@Nullable Object[]>() {
@Override
public Enumerator<@Nullable Object[]> enumerator() {
//返回我们自定义的读取数据的类
return new CsvEnumerator<>(source, cancelFlag, false, null,
CsvEnumerator.arrayConverter(fieldTypes, fields, false));
}
};
}
public CsvEnumerator(Source source, AtomicBoolean cancelFlag, boolean stream,
@Nullable String @Nullable [] filterValues, RowConverter<E> rowConverter){
this.cancelFlag = cancelFlag;
this.rowConverter = rowConverter;
this.filterValues = filterValues == null ? null
: ImmutableNullableList.copyOf(filterValues);
try {
this.reader = openCsv(source);
//跳过第一行,因为第一行是定义类型和名称的
this.reader.readNext(); // skip header row
} catch (IOException e) {
throw new RuntimeException(e);
}
}
//CsvEnumerator必须实现calcit自己的迭代器,里面有current、moveNext方法,current是返回当前游标所在的数据记录,moveNext是将游标指向下一个记录,官网中自己定义了一个类型转换器,是将csv文件中的数据转换成文件头指定的类型,这个需要我们自己来实现
@Override
public E current(){
return castNonNull(current);
}
@Override
public boolean moveNext(){
try {
outer:
for (; ; ) {
if (cancelFlag.get()) {
return false;
}
final String[] strings = reader.readNext();
if (strings == null) {
current = null;
reader.close();
return false;
}
if (filterValues != null) {
for (int i = 0; i < strings.length; i++) {
String filterValue = filterValues.get(i);
if (filterValue != null) {
if (!filterValue.equals(strings[i])) {
continue outer;
}
}
}
}
current = rowConverter.convertRow(strings);
return true;
}
} catch (IOException e) {
throw new RuntimeException(e);
}
}
protected @Nullable Object convert(@Nullable RelDataType fieldType, @Nullable String string){
if (fieldType == null || string == null) {
return string;
}
switch (fieldType.getSqlTypeName()) {
case BOOLEAN:
if (string.length() == 0) {
return null;
}
return Boolean.parseBoolean(string);
case TINYINT:
if (string.length() == 0) {
return null;
}
return Byte.parseByte(string);
case SMALLINT:
if (string.length() == 0) {
return null;
}
return Short.parseShort(string);
case INTEGER:
if (string.length() == 0) {
return null;
}
return Integer.parseInt(string);
case BIGINT:
if (string.length() == 0) {
return null;
}
return Long.parseLong(string);
case FLOAT:
if (string.length() == 0) {
return null;
}
return Float.parseFloat(string);
case DOUBLE:
if (string.length() == 0) {
return null;
}
return Double.parseDouble(string);
case DECIMAL:
if (string.length() == 0) {
return null;
}
return parseDecimal(fieldType.getPrecision(), fieldType.getScale(), string);
case DATE:
if (string.length() == 0) {
return null;
}
try {
Date date = TIME_FORMAT_DATE.parse(string);
return (int) (date.getTime() / DateTimeUtils.MILLIS_PER_DAY);
} catch (ParseException e) {
return null;
}
case TIME:
if (string.length() == 0) {
return null;
}
try {
Date date = TIME_FORMAT_TIME.parse(string);
return (int) date.getTime();
} catch (ParseException e) {
return null;
}
case TIMESTAMP:
if (string.length() == 0) {
return null;
}
try {
Date date = TIME_FORMAT_TIMESTAMP.parse(string);
return date.getTime();
} catch (ParseException e) {
return null;
}
case VARCHAR:
default:
return string;
}
}
6. 最后
至此我们需要准备的东西:库、表名称、字段名称、字段类型都有了,接下来我们去写我们的 SQL 语句查询我们的数据文件。
创建好几个测试的数据文件,例如上面项目结构中我创建 2 个 csv 文件USERINFO.csv
、ASSET.csv
,然后创建测试类。
这样跑起来,就可以通过 SQL 语句的方式直接查询数据了。
public class Test {
public static void main(String[] args) throws SQLException {
Connection connection = null;
Statement statement = null;
try {
Properties info = new Properties();
info.put("model", Sources.of(Test.class.getResource("/model.json")).file().getAbsolutePath());
connection = DriverManager.getConnection("jdbc:calcite:", info);
statement = connection.createStatement();
print(statement.executeQuery("select * from asset "));
print(statement.executeQuery(" select * from userinfo "));
print(statement.executeQuery(" select age from userinfo where name ='aixiaoxian' "));
print(statement.executeQuery(" select * from userinfo where age >60 "));
print(statement.executeQuery(" select * from userinfo where name like 'a%' "));
} finally {
connection.close();
}
}
private static void print(ResultSet resultSet) throws SQLException {
final ResultSetMetaData metaData = resultSet.getMetaData();
final int columnCount = metaData.getColumnCount();
while (resultSet.next()) {
for (int i = 1; ; i++) {
System.out.print(resultSet.getString(i));
if (i < columnCount) {
System.out.print(", ");
} else {
System.out.println();
break;
}
}
}
}
}
查询结果:
这里在测试的时候踩到2个坑,大家如果自己实验的时候可以避免下。
-
Calcite
默认会把你的 SQL 语句中的表名和类名全部转换为大写,因为默认的 csv(其他文件也一样)文件的名称就是表名,除非你自定义规则,所以你的文件名要写成大写。 -
Calcite
有一些默认的关键字不能用作表名,不然会查询失败,比如我刚开始定的user.csv
就一直查不出来,改成USERINFO
就可以了,这点和Mysql
的内置关键字差不多,也可以通过个性化配置去改。
演示Mysql
- 首先,还是先准备
Calcite
需要的东西:库、表名称、字段名称、字段类型。
如果数据源使用Mysql
的话,这些都不用我们去 JAVA 服务中去定义,直接在 Mysql 客户端创建好,这里直接创建两张表用于测试,就和我们的csv
文件一样。
CREATE TABLE `USERINFO1` (
`NAME` varchar(255) CHARACTER SET utf8mb3 COLLATE utf8_general_ci DEFAULT NULL,
`AGE` int DEFAULT NULL
) ENGINE=InnoDB DEFAULT CHARSET=utf8mb3;
CREATE TABLE `ASSET` (
`NAME` varchar(255) CHARACTER SET utf8mb3 COLLATE utf8_general_ci DEFAULT NULL,
`MONEY` varchar(255) CHARACTER SET utf8mb3 COLLATE utf8_general_ci DEFAULT NULL
) ENGINE=InnoDB DEFAULT CHARSET=utf8mb3;
- 上述
csv
案例中的 SchemaFactory
以及 Schema
这些都不需要创建,因为 Calcite
默认提供了 Mysql 的 Adapter适配器。 - 其实,上述两步都不需要做,我们真正要做的是,告诉
Calcite
你的 JDBC 的连接信息就行了,也是在 model.json
文件中定义。
{
"version": "1.0",
"defaultSchema": "Demo",
"schemas": [
{
"name": "Demo",
"type": "custom",
// 这里是calcite默认的SchemaFactory,里面的流程和我们上述自己定义的相同,下面会简单看看源码。
"factory": "org.apache.calcite.adapter.jdbc.JdbcSchema$Factory",
"operand": {
// 我用的是mysql8以上版本,所以这里注意包的名称
"jdbcDriver": "com.mysql.cj.jdbc.Driver",
"jdbcUrl": "jdbc:mysql://localhost:3306/irving",
"jdbcUser": "root",
"jdbcPassword": "123456"
}
}
]
}
- 在项目中引入 Mysql 的驱动包
<dependency>
<groupId>mysql</groupId>
<artifactId>mysql-connector-java</artifactId>
<version>8.0.30</version>
</dependency>
- 写好测试类,这样直接就相当于完成了所有的功能了。
public class TestMysql {
public static void main(String[] args) throws SQLException {
Connection connection = null;
Statement statement = null;
try {
Properties info = new Properties();
info.put("model", Sources.of(TestMysql.class.getResource("/mysqlmodel.json")).file().getAbsolutePath());
connection = DriverManager.getConnection("jdbc:calcite:", info);
statement = connection.createStatement();
statement.executeUpdate(" insert into userinfo1 values ('xxx',12) ");
print(statement.executeQuery("select * from asset "));
print(statement.executeQuery(" select * from userinfo1 "));
print(statement.executeQuery(" select age from userinfo1 where name ='aixiaoxian' "));
print(statement.executeQuery(" select * from userinfo1 where age >60 "));
print(statement.executeQuery(" select * from userinfo1 where name like 'a%' "));
} finally {
connection.close();
}
}
private static void print(ResultSet resultSet) throws SQLException {
final ResultSetMetaData metaData = resultSet.getMetaData();
final int columnCount = metaData.getColumnCount();
while (resultSet.next()) {
for (int i = 1; ; i++) {
System.out.print(resultSet.getString(i));
if (i < columnCount) {
System.out.print(", ");
} else {
System.out.println();
break;
}
}
}
}
}
查询结果:
Mysql实现原理
上述我们在 model.json
文件中指定了org.apache.calcite.adapter.jdbc.JdbcSchema$Factory
类,可以看下这个类的代码。
这个类是把 Factory
和 Schema
写在了一起,其实就是调用schemafactory
类的create
方法创建一个 schema
出来,和我们上面自定义的流程是一样的。
其中JdbcSchema
类也是 Schema
的子类,所以也会实现getTable
方法(这个我们上述也实现了,我们当时是获取表结构和表的字段类型以及名称,是从csv文件头中读文件的),JdbcSchema
的实现是通过连接 Mysql 服务端查询元数据信息,再将这些信息封装成 Calcite
需要的对象格式。
这里同样要注意 csv
方式的2个注意点,大小写和关键字问题。
public static JdbcSchema create(
SchemaPlus parentSchema,
String name,
Map<String, Object> operand){
DataSource dataSource;
try {
final String dataSourceName = (String) operand.get("dataSource");
if (dataSourceName != null) {
dataSource =
AvaticaUtils.instantiatePlugin(DataSource.class, dataSourceName);
} else {
//会走在这里来,这里就是我们在model.json中指定的jdbc的连接信息,最终会创建一个datasource
final String jdbcUrl = (String) requireNonNull(operand.get("jdbcUrl"), "jdbcUrl");
final String jdbcDriver = (String) operand.get("jdbcDriver");
final String jdbcUser = (String) operand.get("jdbcUser");
final String jdbcPassword = (String) operand.get("jdbcPassword");
dataSource = dataSource(jdbcUrl, jdbcDriver, jdbcUser, jdbcPassword);
}
} catch (Exception e) {
throw new RuntimeException("Error while reading dataSource", e);
}
String jdbcCatalog = (String) operand.get("jdbcCatalog");
String jdbcSchema = (String) operand.get("jdbcSchema");
String sqlDialectFactory = (String) operand.get("sqlDialectFactory");
if (sqlDialectFactory == null || sqlDialectFactory.isEmpty()) {
return JdbcSchema.create(
parentSchema, name, dataSource, jdbcCatalog, jdbcSchema);
} else {
SqlDialectFactory factory = AvaticaUtils.instantiatePlugin(
SqlDialectFactory.class, sqlDialectFactory);
return JdbcSchema.create(
parentSchema, name, dataSource, factory, jdbcCatalog, jdbcSchema);
}
}
@Override public @Nullable Table getTable(String name){
return getTableMap(false).get(name);
}
private synchronized ImmutableMap<String, JdbcTable> getTableMap(
boolean force){
if (force || tableMap == null) {
tableMap = computeTables();
}
return tableMap;
}
private ImmutableMap<String, JdbcTable> computeTables(){
Connection connection = null;
ResultSet resultSet = null;
try {
connection = dataSource.getConnection();
final Pair<@Nullable String, @Nullable String> catalogSchema = getCatalogSchema(connection);
final String catalog = catalogSchema.left;
final String schema = catalogSchema.right;
final Iterable<MetaImpl.MetaTable> tableDefs;
Foo threadMetadata = THREAD_METADATA.get();
if (threadMetadata != null) {
tableDefs = threadMetadata.apply(catalog, schema);
} else {
final List<MetaImpl.MetaTable> tableDefList = new ArrayList<>();
// 获取元数据
final DatabaseMetaData metaData = connection.getMetaData();
resultSet = metaData.getTables(catalog, schema, null, null);
while (resultSet.next()) {
//获取库名,表明等信息
final String catalogName = resultSet.getString(1);
final String schemaName = resultSet.getString(2);
final String tableName = resultSet.getString(3);
final String tableTypeName = resultSet.getString(4);
tableDefList.add(
new MetaImpl.MetaTable(catalogName, schemaName, tableName,
tableTypeName));
}
tableDefs = tableDefList;
}
final ImmutableMap.Builder<String, JdbcTable> builder =
ImmutableMap.builder();
for (MetaImpl.MetaTable tableDef : tableDefs) {
final String tableTypeName2 =
tableDef.tableType == null
? null
: tableDef.tableType.toUpperCase(Locale.ROOT).replace(' ', '_');
final TableType tableType =
Util.enumVal(TableType.OTHER, tableTypeName2);
if (tableType == TableType.OTHER && tableTypeName2 != null) {
System.out.println("Unknown table type: " + tableTypeName2);
}
// 最终封装成JdbcTable对象
final JdbcTable table =
new JdbcTable(this, tableDef.tableCat, tableDef.tableSchem,
tableDef.tableName, tableType);
builder.put(tableDef.tableName, table);
}
return builder.build();
} catch (SQLException e) {
throw new RuntimeException(
"Exception while reading tables", e);
} finally {
close(connection, null, resultSet);
}
}
SQL执行流程
OK,到这里基本上两个简单的案例已经演示好了,最后补充一下整个Calcite
架构和整个 SQL 的执行流程。
整个流程如下:SQL解析(Parser)=> SQL校验(Validator)=> SQL查询优化(optimizer)=> SQL生成 => SQL执行
SQL Parser
所有的 SQL 语句在执行前都需要经历 SQL 解析器解析,解析器的工作内容就是将 SQL 中的 Token 解析成抽象语法树,每个树的节点都是一个 SqlNode,这个过程其实就是 Sql Text => SqlNode 的过程。
我们前面的 Demo 没有自定义 Parser,是因为 Calcite 采用了自己默认的 Parser(SqlParserImpl)。
SqlNode
SqlNode
是整个解析中的核心,比如图中你可以发现,对于每个比如select
、from
、where
关键字之后的内容其实都是一个SqlNode
。
parserConfig
方法主要是设置 SqlParserFactory 的参数,比如我们上面所说的我本地测试的时候踩的大小写的坑,就可以在这里设置。
直接调用setCaseSensitive=false
即不会将 SQL 语句中的表名列名转为大写,下面是默认的,其他的参数可以按需配置。
SQL Validator
SQL 语句先经过 Parser,然后经过语法验证器,注意 Parser 并不会验证语法的正确性。
其实 Parser 只会验证 SQL 关键词的位置是否正确,我们上述2个 Parser 的例子中都没有创建 schema
和 table
这些,但是如果这样写,那就会报错,这个错误就是 parser
检测后抛出来的(ParseLocationErrorTest)。
真正的校验在 validator
中,会去验证查询的表名是否存在,查询的字段是否存在,类型是否匹配,这个过程比较复杂,默认的 validator
是SqlValidatorImpl
。
查询优化
比如关系代数,比如什么投影、笛卡尔积这些,Calcite
提供了很多内部的优化器,也可以实现自己的优化器。
适配器
Calcite
是不包含存储层的,所以提供一种适配器的机制来访问外部的数据存储或者存储引擎。
最后,进阶
官网里面写了未来会支持Kafka
适配器到公共Api
中,到时候使用起来就和上述集成Mysql
一样方便,但是现在还没有支持,我这里给大家提供个自己实现的方式,这样就可以通过 SQL 的方式直接查询 Kafka 中的 Topic 数据等信息。
这里我们内部集成实现了KSQL
的能力,查询结果是OK的。
还是像上述步骤一样,我们需要准备库、表名称、字段名称、字段类型、数据源(多出来的地方)。
- 自定义
Sql
解析,之前我们都没有自定义解析,这里需要自定义解析,是因为我需要动态解析sql
的where
条件里面的partation
。
- 配置解析器,就是之前案例中提到的配置大小写之类的
- 创建解析器,使用的默认
SqlParseImpl
- 开始解析,生成
AST
,我们可以基于生成的SqlNode
做一些业务相关的校验和参数解析
- 适配器获取数据源
public class KafkaConsumerAdapter {
public static List<KafkaResult> executor(KafkaSqlInfo kafkaSql){
Properties props = new Properties();
props.put(CommonClientConfigs.BOOTSTRAP_SERVERS_CONFIG, kafkaSql.getSeeds());
props.put(ConsumerConfig.KEY_DESERIALIZER_CLASS_CONFIG, StringDeserializer.class.getCanonicalName());
props.put(ConsumerConfig.VALUE_DESERIALIZER_CLASS_CONFIG, StringDeserializer.class.getCanonicalName());
props.put(ConsumerConfig.AUTO_OFFSET_RESET_CONFIG, "earliest");
KafkaConsumer<String, String> consumer = new KafkaConsumer<>(props);
List<TopicPartition> topics = new ArrayList<>();
for (Integer partition : kafkaSql.getPartition()) {
TopicPartition tp = new TopicPartition(kafkaSql.getTableName(), partition);
topics.add(tp);
}
consumer.assign(topics);
for (TopicPartition tp : topics) {
Map<TopicPartition, Long> offsets = consumer.endOffsets(Collections.singleton(tp));
long position = 500;
if (offsets.get(tp).longValue() > position) {
consumer.seek(tp, offsets.get(tp).longValue() - 500);
} else {
consumer.seek(tp, 0);
}
}
List<KafkaResult> results = new ArrayList<>();
boolean flag = true;
while (flag) {
ConsumerRecords<String, String> records = consumer.poll(Duration.ofMillis(100));
for (ConsumerRecord<String, String> record : records) {
//转成我定义的对象集合
KafkaResult result = new KafkaResult();
result.setPartition(record.partition());
result.setOffset(record.offset());
result.setMsg(record.value());
result.setKey(record.key());
results.add(result);
}
if (!records.isEmpty()) {
flag = false;
}
}
consumer.close();
return results;
}
}
- 执行查询,就可以得到我们想要的效果了。
public class TestKafka {
public static void main(String[] args) throws Exception {
KafkaService kafkaService = new KafkaService();
//把解析到的参数放在我自己定义的kafkaSqlInfo对象中
KafkaSqlInfo sqlInfo = kafkaService.parseSql("select * from `cmdb-calltopo` where `partition` in (0,1,2) limit 1000 ");
//适配器获取数据源,主要是从上述的sqlInfo对象中去poll数据
List<KafkaResult> results = KafkaConsumerAdapter.executor(sqlInfo);
//执行查询
query(sqlInfo.getTableName(), results, sqlInfo.getSql());
sqlInfo = kafkaService.parseSql("select * from `cmdb-calltopo` where `partition` in (0,1,2) AND msg like '%account%' limit 1000 ");
results = KafkaConsumerAdapter.executor(sqlInfo);
query(sqlInfo.getTableName(), results, sqlInfo.getSql());
sqlInfo = kafkaService.parseSql("select count(*) AS addad from `cmdb-calltopo` where `partition` in (0,1,2) limit 1000 ");
results = KafkaConsumerAdapter.executor(sqlInfo);
query(sqlInfo.getTableName(), results, sqlInfo.getSql());
}
private static void query(String tableName, List<KafkaResult> results,
String sql) throws Exception {
//创建model.json,设置我的SchemaFactory,设置库名
String model = createTempJson();
//设置我的表结构,表名称和表字段名以及类型
KafkaTableSchema.generateSchema(tableName, results);
Properties info = new Properties();
info.setProperty("lex", Lex.JAVA.toString());
Connection connection = DriverManager.getConnection(Driver.CONNECT_STRING_PREFIX + "model=inline:" + model, info);
Statement st = connection.createStatement();
//执行
ResultSet result = st.executeQuery(sql);
ResultSetMetaData rsmd = result.getMetaData();
List<Map<String, Object>> ret = new ArrayList<>();
while (result.next()) {
Map<String, Object> map = new LinkedHashMap<>();
for (int i = 1; i <= rsmd.getColumnCount(); i++) {
map.put(rsmd.getColumnName(i), result.getString(rsmd.getColumnName(i)));
}
ret.add(map);
}
result.close();
st.close();
connection.close();
}
private static void print(ResultSet resultSet) throws SQLException {
final ResultSetMetaData metaData = resultSet.getMetaData();
final int columnCount = metaData.getColumnCount();
while (resultSet.next()) {
for (int i = 1; ; i++) {
System.out.print(resultSet.getString(i));
if (i < columnCount) {
System.out.print(", ");
} else {
System.out.println();
break;
}
}
}
}
private static String createTempJson() throws IOException {
JSONObject object = new JSONObject();
object.put("version", "1.0");
object.put("defaultSchema", "QAKAFKA");
JSONArray array = new JSONArray();
JSONObject tmp = new JSONObject();
tmp.put("name", "QAKAFKA");
tmp.put("type", "custom");
tmp.put("factory", "kafka.KafkaSchemaFactory");
array.add(tmp);
object.put("schemas", array);
return object.toJSONString();
}
}
- 生成临时的
model.json
,之前是基于文件,现在基于text
字符串,mode=inline
模式 - 设置我的表结构、表名称、字段名、字段类型等,并放置在内存中,同时将适配器查询出来的数据也放进去
table
里面 - 获取连接,执行查询,完美!
文章转载自公众号:艾小仙
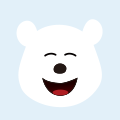