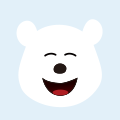
深度干货|PolarDB分布式版基于向量化SIMD指令的探索
4. 表达式计算
Long数组相加
Vector API的最大优势就是加速计算,因此接下来我们会探索其可能能够带来性能提升的场景。首先我们对前文中给出的Long数组相加的场景进行了Benchmark,可以看到在数组相加场景下标量执行和SIMD执行相差不大,通过对汇编指令的追踪,我们发现不论是SIMD执行还是标量执行最终都会生成vpaddq这条指令:
1. SIMD执行
0x00007f3eb13602ae: vpaddq 0x10(%r11,%rbx,8),%ymm3,%ymm3;
2. 标量执行
0x00007fa6fd33259a: vpaddq 0x10(%r8,%rbp,8),%ymm4,%ymm4
vpaddq:AVX512指令集。将(%r11 + %rbx * 8)开始的16个字节的数据和ymm3寄存器中的数据相加,写到ymm3寄存器中。
自动向量化(auto-vectorization)
我们发现,哪怕没有显示的使用Vector API,向量化加法的代码也会被进行向量化,这源于Java的自动向量化(auto-vectorization)机制。Java自动向量化的实现与Vector API类似,其会在JIT编译的时候检查代码能否使用SIMD指令进行运算,如果可以即替换为SIMD实现。但是自动向量化仅能处理比较简单的计算,对于复杂计算仍然需要手动SIMD(使用Vector API)。例如自动向量化已知的限制有:
1. 只支持自增的for循环
2. 只支持Int/Long类型(Short/Byte/Char 通过int间接支持)
3. 循环的上限必须是常量
通过对一些成熟OLAP系统(例如ClickHouse)的调研,以及我们线上实际场景的探索,我们使用Vector API重新实现了一批算子,这里我们将会展示4种比较有代表性的场景。
5. 大小写转化
使用SIMD实现大小写转化的思路比较简单,我们只需要调用compare方法进行比较,使用lanewise方法进行异或即可。这里引入了VectorMask,可以理解为一个boolean类型的寄存器,里面含有n个0/1。
@Benchmark
public void normal() {
final long Mask = 'A' ^ 'a';
for (int i = 0; i < charArr.length; i++) {
if(charArr[i] >= 'A' && charArr[i] <= 'Z') {
charArr[i] ^= Mask;
}
}
}
@Benchmark
public void vector() {
final long Mask = 'A' ^ 'a';
int i;
int batchSize = charArr.length;
int end = SPECIES.loopBound(charArr.length);
for (i = 0; i < end; i += SPECIES.length()) {
ByteVector from = ByteVector.fromArray(SPECIES, charArr, i);
VectorMask mask = from.compare(VectorOperators.GE, 'A', from.compare(VectorOperators.LE, 'Z'));
ByteVector to = from.lanewise(VectorOperators.XOR, Mask, mask); //这里传入了mask,表示只对mask=1的位置执行xor
to.intoArray(charArr, i);
}
for(; i < batchSize; ++i) {
if(charArr[i] >= 'A' && charArr[i] <= 'Z') {
charArr[i] ^= Mask;
}
}
}
Benchmark
测试环境:随机生成1000和10w个byte类型的字母来进行Benchmark
测试结果:在count=10w的场景下快了50x,原因在于ByteSpecies的长度为32,同时SIMD的执行方式没有分支预测失败flush流水线的开销。
6. SIMD Filter
使用SIMD指令实现Filter可以使用Gather运算读取数组中的元素,并使用compare方法进行比较,最后采用位运算的方式记录下满足条件的下标。
@Benchmark
public void normal() {
newSize = 0;
for (int i = 0; i < intArr.length; i++) {
if(intArr[sel[i]] <= intArr2[sel[i]) {
sel[newSize++] = i;
}
}
}
@Benchmark
public void vector() {
newSize = 0;
int i;
int batchSize = intArr.length;
int end = SPECIES.loopBound(intArr.length);
for (i = 0; i < end; i += SPECIES.length()) {
IntVector va = IntVector.fromArray(SPECIES, intArr, 0, sel, i);
IntVector vb = IntVector.fromArray(SPECIES, intArr2, 0, sel, i);
VectorMask<Integer> vc = va.compare(VectorOperators.LE, vb);
if(!vc.anyTrue()) {
continue;
}
int res = (int) vc.toLong();
while(res != 0) {
int last = res & -res; //找到二进制中最后一个1对应的幂次, 例如12 = 1100, 12 & (-12) = 4
sel[newSize++] = i + Integer.numberOfTrailingZeros(last); //numberOfTrailingZeros(2^i) = i
res ^= last;
}
}
for(; i < batchSize; ++i) {
int j = sel[i];
if(intArr[j] <= intArr2[j]) {
sel[newSize++] = i;
}
}
}
Benchmark
测试环境:随机生成1000和10w个int类型的整数来进行Benchmark
结果分析:在count=1000时SIMD的性能反而下降,这是因为此时函数并没有JIT,而是采用了JDK层面的标量执行。在count=10w时方法已经被JIT,因此性能会有25%的提升。
7. Local Exchange
什么是Local Exchange算子
Exchange算子是PolarDB分布式版MPP执行模式中进行数据shuffle的重要组件,关于其背景可以参考文章《PolarDB-X并行计算框架》[3],这里不再赘述。在这里,我们主要来优化Local Exchange算子中的LocalPartitionExchanger执行模式。本次实践中,我们通过向量化算子 + SIMD Scatter指令的方式实现了35%的性能提升。
LocalPartitionExchanger算子可以简单理解为: 对于某个下游pipline中的driver,其会对Chunk中的每行数据计算对应的partition分区,并将相同partition分区的数据重新组装为一个新的Chunk喂给上游driver。
对于这一段表述不理解的话也没关系,本文的重点在于介绍使用SIMD指令优化代码逻辑的方式,因此可以放心继续阅读下文。
非向量化版本(PolarDB分布式版现有版本)
PolarDB分布式版现有版本沿用了行存执行模型下的Local Exchange算子,其基本思想是row by row的逐行枚举、逐行计算partition并写入对应的Chunk,这一执行模式的缺点在于其既不能有效的利用列式内存布局,同时appendTo操作会带来大量的时间开销。
旧版本的详细执行流程为:
1. 计算position(行号)对应的partition:使用了n个链表来保存每个partition对应的position list
a. (partitionGenerator.getPartition可以简单的理解为对position对应的数据进行hash运算得到目标partition的位置)
for (int position = 0; position < keyChunk.getPositionCount(); position++) {
int partition = partitionGenerator.getPartition(keyChunk, position);
partitionAssignments[partition].add(position);
}
2. buildChunk:生成对应partition的Chunk
a. 先枚举partition
i. 枚举partition对应的position(position表示行)
1. 枚举该行对应的列block
a. 调用builder的appendTo来为ChunkBuilder添加元素
b. appendTo有一次虚函数调用,性能开销极大!
Map<Integer, Chunk> partitionChunks = new HashMap<>();
for (int partition = 0; partition < executors.size(); partition++) { //枚举partition
List<Integer> positions = partitionAssignments[partition]; //获取到对应的positions = partitionAssignments[partition]
ChunkBuilder builder = new ChunkBuilder(types, positions.size(), context);
Chunk partitionedChunk;
for (Integer pos : positions) { //枚举对应的position(行)
for (int i = 0; i < chunk.getBlockCount(); i++) { //枚举对应的列
builder.appendTo(chunk.getBlock(i), i, pos); //将pos行,i列位置的元素添加到对应的ChunkBuilder
}
}
partitionedChunk = builder.build();
partitionChunks.put(partition, partitionedChunk);
}
Local Exchange SIMD优化
思路:
1. 对appendTo的优化: appendTo操作开销极大,尝试用更高效的方法拷贝数据, 例如system.arrayCopy
2. 对行式枚举模式的优化:逐行枚举的方式受限,因为每一列的数据在不同的Block内,不可能攒批copy。同时逐行枚举的方式对访存不友好。那么考虑先枚举列。
● 问题: 每一个partition对应的行并不连续;
● 解决方案:如下图所示,我们希望求出positionMapping数组使得相同partition的行能够被迁移到连续的行中,这样以来就可以使用Scatter运算进行加速。
afterValue[positionMapping[i]]
3. 问题:如何知道positionMapping?
● 其实很简单,线性扫描一遍,记录下每个partition的size, 记为partitoinSize[]
● 知道了size,就可以求出每个partition在最终数组中的偏移量paritionOffset[]
● 已知offset就可以边遍历边求出positionMapping:只需要记录出该行对应的partition的offset,然后让offset自增即可。这一过程看代码会更直观。
具体步骤
1. 计算positionMapping[]
● 计算每个位置对应的partition以及每个partition的size
private void scatterAssignmentNoneBucketPageInBatch(Chunk keyChunk, int positionStart, int batchSize) {
int[] partitions = scatterMemoryContext.getPartitionsBuffer();
for (int i = 0; i < batchSize; i++) {
int partition = partitionGenerator.getPartition(keyChunk, i + positionStart);
partitions[i] = partition;
++paritionSize[partition]; //统计每个partition的size
}
}
● 计算每个partition对应的offset
private void prepareDestScatterMapStartOffset() {
int startOffset = 0;
for (int i = 0; i < partitionNum; i++) {
partitionOffset[i] = startOffset; //统计每个partition对应的偏移量
startOffset += partitionSize[i];
}
}
● 计算positionMapping
protected void prepareLengthsPerPartitionInBatch(int batchSize, int[] partitions, int[] destScatterMap) {
for (int i = 0; i < batchSize; ++i) {
positionMapping[i] = partitionOffset[partitions[i]]++; //获取每个位置scatter之后对应的内存地址
}
}
2. 使用scatter运算
● vectorizedSIMDScatterAppendTo中会判断该Block是否支持SIMD执行,并调用Block的copyPositions_scatter_simd方法,这里以IntBlock为例:
protected void scatterCopyNoneBucketPageInBatch(Chunk keyChunk, int positionStart, int batchSize) {
Block[] sourceBlocks = keyChunk.getBlocksDirectly(); //获取到所有的block
for (int col = 0; col < types.size(); col++) { //枚举所有列
Block sourceBlock = sourceBlocks[col]; //获取到block
for (int i = 0; i < chunkBuilders.length; i++) {
blockBuilders[i] = chunkBuilders[i].getBlockBuilder(col); //获取到所有partition的pageBuilder
}
vectorizedSIMDScatterAppendTo(sourceBlock, scatterMemoryContext, blockBuilders);
}
}
public void copyPositions_scatter_simd(ScatterMemoryContext scatterMemoryContext, BlockBuilder[] blockBuilders) {
//存放目的数据的buffer
int[] afterValue = scatterMemoryContext.getafterValue();
//存放原始数据的buffer
int[] preValue = scatterMemoryContext.getpreValue();
//positionMapping
int[] positionMapping = scatterMemoryContext.getpositionMapping();
//每个partition的size
int[] partitionSize = scatterMemoryContext.getpartitionSize();
//使用scatter的操作
VectorizedPrimitives.SIMD_PRIMITIVES_HANDLER.scatter(preValue, afterValue, positionMapping, 0, batchSize);
int start = 0;
for (int partition = 0; partition < blockBuilders.length; partition++) {
IntegerBlockBuilder unsafeBlockBuilder = (IntegerBlockBuilder) blockBuilders[partition];
//注意这里writeBatchInts的实现
unsafeBlockBuilder.writeBatchInts(afterValue, bufferNullsBuffer, start, partitionSize[partition]);
start += partitionSize[partition];
}
}
3. 使用writeBatchInts来实现内存拷贝
public IntegerBlockBuilder writeBatchInts(int[] ints, boolean[] nulls, int sourceIndex, int count) {
values.addElements(getPositionCount(), ints, sourceIndex, count);
valueIsNull.addElements(getPositionCount(), nulls, sourceIndex, count);
return this;
}
addElements的底层使用了System.arraycopy命令来批量的进行内存复制 System.arraycopy是JVM的内置函数,其实现效率远远快于调用append接口来逐个添加数据。
@IntrinsicCandidate
public static native void arraycopy(Object src, int srcPos,
Object dest, int destPos,
int length);
Benchmark
测试环境:我们设置parition的数量为3,并向Local Exchange算子输入100个大小为1024,包含4列int的Chunk来进行Benchmark
结果分析:
我们发现单纯的向量化算法并不会有性能提升,原因在于Local Exchange算子的瓶颈在于appendTo操作。而SIMD Scatter + System.arrayCopy的方式实现了35%的性能提升。
8. SIMD Hash Join
SIMD思路
在CMU 15-721中提到了SIMD Hash Probe的实现,我们将用Vector API来复现这一过程。
首先来回顾开放地址法的SIMD Hash Probe过程,其基本思路是一次性对4个位置的元素进行probe。
1. 计算出4个位置的hash值
2. 通过Gather运算读取到hash表中对应位置的元素
3. 通过SIMD的compare运算比较有无hash冲突
4. 前3步都比较好理解,接下来我们需要让有hash冲突的元素寻址到下一个位置继续解hash冲突,这一步可以由SIMD加法来实现。重点在于如何让没有冲突的元素移走,把数组中后面的元素放进来继续匹配呢?(例如我们希望把下图中的k1, k4分别替换为k5, k6)
解决方案:
a. 使用expand运算来进行selective load运算
buildPositionVec = buildPositionVec.expand(probePosition, probeOffset, probeMask);
b. expand运算可以将probeMask当中为1的n个位置元素替换为hashPosition数组从probeOffset位置开始的n个元素。
1. 例如在上面的例子中数据的变化为
2. hashPosition = [h1, h2, h3, h4, h5, h6, h7, h8.....]
3. probeOffset = 4
4. probeMask = [1, 0, 0, 1]
5. 入参: [h1, h2, h3, h4]
6. 出参: [h5, h2, h3, h6]
小插曲: 如何实现更强大的expand
在上述代码中,我们的expand运算传入了3个参数,但是openJDK官方的expand函数最多有一个入参(openJDK的expand用法不能传入数组,只能在Vector之间做expand)
这得益于阿里云强大的自研能力,我们与阿里云JVM团队进行了积极的沟通,JVM团队的同学帮助我们实现了更丰富的expand的运算。
验证
PolarDB分布式版的Hash方式并不是开放寻址法,而是布谷鸟Hash,但其SIMD的原理类似,这里给出对布谷鸟Hash的SIMD Hash Probe改造过程。
@Benchmark
public void normal() {
for(int i = 0; i < count; i++) {
int joinedPosition = 0;
int matchedPosition = (int) hashTable.keys[hashedProbeKey[i]];
while (matchedPosition != LIST_END) {
if (buildKey[matchedPosition] == probeKey[i]) {
joinedPosition = matchedPosition;
break;
}
matchedPosition = (int) positionLinks[matchedPosition];
}
joinedPositions[i] = joinedPosition;
}
}
@Benchmark
public void vector() {
int probeOffset = 0;
VectorMask<Long> probeMask;
VectorMask<Integer> intProbeMask;
LongVector probeValueVec = LongVector.fromArray(LongVector.SPECIES_512, probeKey, probeOffset);
// step 1: 使用Gather运算从hashTable的keys中读取数据. int matchedPosition = hashTable.keys[hashedProbeKey[i]];
LongVector buildPositionVec = LongVector.fromArray(LongVector.SPECIES_512, hashTable.keys, 0, hashedProbeKey, probeOffset);
IntVector probeIndexVec = IntVector.fromArray(IntVector.SPECIES_256, index, probeOffset);
probeOffset += 8;
while(probeOffset + LongVector.SPECIES_512.length() < count){
// step 2: 计算buildPositionVec中为0的位置单独处理
VectorMask<Long> emptyMask = buildPositionVec.compare(VectorOperators.EQ, 0);
// step 3: 使用Gather运算计算出buildKey[matchedPosition[i]]的值
IntVector intbuildPositionVec = buildPositionVec.castShape(IntVector.SPECIES_256, 0).reinterpretAsInts();
LongVector buildValueVec = LongVector.fromArray(LongVector.SPECIES_512, intbuildPositionVec, buildKey);
// step 4: 使用SIMD compare来进行比较. if (buildKey[matchedPosition] == probeKey[i])
VectorMask<Long> valueEQMask = probeValueVec.compare(VectorOperators.EQ, buildValueVec, emptyMask.not());
// step 5: 计算出probe过程成功找到目标位置的Mask
probeMask = valueEQMask.or(emptyMask);
intProbeMask = probeMask.cast(IntVector.SPECIES_256);
// step 6: 使用scatter运算将匹配的position写入joinedPosition. joinedPositions[i] = matchedPosition;
buildPositionVec.intoArray(joinedPositions, probeIndexVec, valueEQMask);
// step 7: 处理hash冲突. matchedPosition = positionLinks[matchedPosition];
buildPositionVec = LongVector.fromArray(LongVector.SPECIES_512, intbuildPositionVec, positionLinks);
// step 8: 使用expand运算获取到下一个probe vector
buildPositionVec = buildPositionVec.expand(probePosition, probeOffset, probeMask);
probeValueVec = probeValueVec.expand(probeKey, probeOffset, probeMask);
probeIndexVec = probeIndexVec.expand(index, probeOffset, intProbeMask);
// step 9: 更新probeOffset
probeOffset += probeMask.trueCount();
}
scalarProcessVector(probeValueVec, buildPositionVec, probeIndexVec, joinedPositions); //处理最后一个Vector
if (probeOffset < count) {
processBatchX(hashedProbeKey, probeOffset, count, joinedPositions);
}
}
Benchmark
测试环境:在Build端我们向Hash表中插入了100w个大小在0-1000范围的元素来模拟Hash冲突,Probe时计算探测100w个元素所需要的时间。
测试结果:虽然我们实现了SIMD Hash Probe,但由于现有的Vector API对类型的支持并不充分(代码中含有大量的类型转化),因此实测结果并不优秀,甚至有2倍多的性能下降。
但抛开Java Vector API本身带来的性能下降,使用SIMD指令来优化Hash Probe也许并不是明智之举,这是因为Hash Join的瓶颈不在于计算,而在于访存。《Improving hash join performance through prefetching》写到数据库的Hash Join算法有73%的开销在CPU cache miss上,这也解释了SIMD指令没有优化的原因。
在PolarDB分布式版内部对TPC-H Q9的测试中发现浪费在cache miss上的CPU Cycle达到了计算的10倍之多,因此我们将对Hash Join的优化转移到了cache miss。PolarDB-X已经尝试使用prefetch指令预取(由阿里云JVM团队提供对Java的增强),向量化Hash Probe等方式优化cache miss,相关的优化成果会在后续的文章中展示。
9. 总结
本篇文章我们首先介绍了Vector API的用法与实现原理,并着重探索了其在数据库场景下的应用,在以计算为瓶颈的大小写转化中实现了50倍的性能提升,在Filter算子中实现了25%的性能提升,在Local Exchange算子中实现了35%的性能提升。同时我们也讨论了Vector API在Hash Probe这种以cache miss为瓶颈的算子中的局限性。
云原生数据库PolarDB分布式版作为一款分布式HTAP数据库,AP引擎的性能优化一直是我们的重点工作内容。我们不仅仅着眼于业内的常见优化,对于行列混存架构、向量化SIMD指令等无人涉及的“深水区”也在积极的探索当中。
敬请期待后续PolarDB分布式版列存引擎在公有云和开源的正式发布。
参考文章:
[1] PolarDB-X 向量化执行引擎:https://zhuanlan.zhihu.com/p/337574939
[2] PolarDB-X 向量化引擎:https://zhuanlan.zhihu.com/p/339514444
[3] PolarDB-X 并行计算框架:https://zhuanlan.zhihu.com/p/346320114
文章转载自公众号:阿里云瑶池数据库
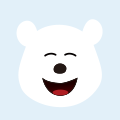